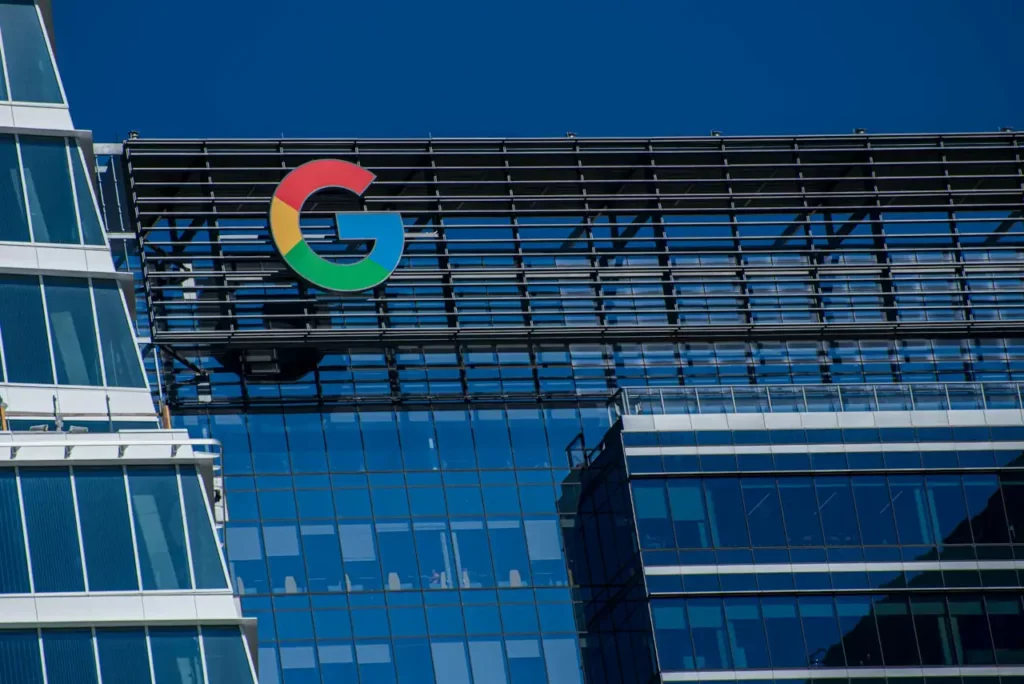
On May 20, 2025, Google DeepMind officially unveiled Veo 3, the newest breakthrough in AI-powered video generation technology. This advanced model represents a significant leap forward in creating high-resolution cinematic video content paired with synchronized, realistic audio—all generated by artificial intelligence. Veo 3’s launch marks a pivotal moment in synthetic media, opening new possibilities across entertainment, marketing, education, and many other sectors while raising important questions about the future of content creation.
In this article, we explore Veo 3 in detail—from its technical underpinnings and theoretical significance to practical applications, business implications, development journey, and ethical considerations. Drawing from publicly available data and the history of DeepMind’s research, this analysis aims to provide a comprehensive, accurate, and professional overview of one of 2025’s most exciting AI advances.
The Genesis of Deepmind Veo 3: Background and Development Journey
Google DeepMind, founded in 2010 and acquired by Google in 2015, has long been a pioneer in artificial intelligence research. Known for groundbreaking achievements such as AlphaGo’s defeat of the world’s top Go players and AlphaFold’s revolution in protein folding prediction, DeepMind has expanded its focus to include multimodal AI capable of understanding and generating complex audiovisual data.
The Veo series of models began as DeepMind’s effort to generate video content through machine learning. Early iterations focused primarily on short, low-resolution clips, struggling with issues of temporal consistency and lack of audio integration. Veo 2 marked a significant improvement by incorporating some audio conditioning but remained limited in resolution and coherence.
Veo 3 is the culmination of years of research involving a multidisciplinary team of computer vision scientists, audio engineers, machine learning researchers, and software engineers. The team’s work was supported by advances in transformer architectures, large-scale datasets, and TPU-based distributed training. According to DeepMind’s research publications and public statements, Veo 3 was trained on over 20 million hours of video data and audio, sourced from licensed datasets and publicly available media, ensuring diversity across genres and contexts.
The project spanned over three years of iterative development, with a strong focus on overcoming prior limitations related to temporal stability and realistic audio-visual alignment. The research team introduced novel methods in hierarchical video synthesis and joint audio-visual latent space modeling, enabling Veo 3 to generate videos with unprecedented fidelity and naturalism.
Technical Architecture: The Heart of Deepmind Veo 3
Veo 3’s architecture builds on transformer-based neural networks, which have revolutionized natural language processing and are now at the forefront of generative models for images, video, and audio.
The core innovation lies in Veo 3’s multimodal transformer backbone, a single neural network that processes and generates both video frames and audio waveforms simultaneously. This contrasts with traditional systems that separate visual and audio generation into isolated pipelines, often resulting in disjointed outputs.
The video generation process begins with a low-resolution “base” frame sequence, which is progressively refined through multiple stages using hierarchical upsampling techniques. This multiscale approach efficiently manages computational resources while maintaining fine details necessary for cinematic quality, reaching up to 4K resolution at 60 frames per second.
Audio synthesis in Veo 3 is conditioned on the visual context, using cross-attention mechanisms within the transformer to ensure sounds align precisely with corresponding actions, speech, and environmental cues. For example, dialogue generated in the video is matched with lip movements and emotional tone, while ambient sounds respond dynamically to scene changes.
To maintain temporal consistency across frames, Veo 3 incorporates recurrent neural network (RNN) modules alongside transformers, allowing the model to track motion trajectories and lighting shifts over extended sequences. This innovation addresses a major challenge in generative video AI—avoiding flickering artifacts and unrealistic transitions.
The training regimen combined contrastive learning to better associate audio-visual pairs, self-supervised pretraining to learn general representations from unlabeled data, and adversarial training to improve realism by pitting the generator against discriminators trained to detect synthetic outputs.
DeepMind’s research indicates that Veo 3’s model size exceeds 15 billion parameters, positioning it among the largest multimodal AI systems to date. This scale contributes to its nuanced understanding of audiovisual contexts but also demands substantial computational power, running on clusters of Google’s custom TPUs over weeks of training.
Dataset and Training: Fueling the AI Engine
The scale and diversity of training data underpin Veo 3’s capabilities. According to DeepMind, the model was trained on over 20 million hours of video content and corresponding audio streams from a wide array of sources, including licensed cinema footage, documentaries, sports broadcasts, and user-generated content.
Metadata associated with these videos—such as scene descriptions, object labels, dialogue transcripts, and environmental tags—allowed the model to learn complex correlations across modalities and contexts.
Importantly, the dataset was curated to minimize biases and to represent a variety of ethnicities, languages, environments, and genres, reflecting DeepMind’s commitment to ethical AI development. Despite these efforts, researchers acknowledge that challenges remain in fully eliminating dataset-induced biases.
The distributed training used Google’s TPU v5 pods, enabling parallel processing of enormous datasets and model parameters. The training cycle spanned approximately three months, a reflection of the scale and complexity involved.
Theoretical Contributions: Advancing Multimodal AI
Veo 3 represents a landmark theoretical advance in how AI models understand and generate multisensory data. Previous AI systems often handled modalities such as vision and sound separately, limiting the naturalism and coherence of synthesized outputs.
By employing a unified multimodal transformer, Veo 3 learns a joint latent space where audio and visual features coexist and influence one another. This enables the model to predict and generate audio from visual context and vice versa, providing a richer understanding of the scene dynamics.
This approach contributes to the growing field of cross-modal learning, which has implications far beyond video generation, including robotics, human-computer interaction, and augmented reality. Understanding how to represent and integrate multisensory data at scale is a fundamental challenge in AI, and Veo 3 offers valuable insights and methodologies.
Moreover, Veo 3’s hierarchical video generation introduces a scalable framework to synthesize long, high-resolution sequences efficiently—a problem that has limited previous research. The inclusion of temporal modeling mechanisms like recurrent layers further advances the ability to capture complex dynamics over time, a key factor in achieving cinematic quality.
Practical Applications: Transforming Creative Industries and Beyond
The potential applications of Veo 3 are expansive. In entertainment, the model offers filmmakers and content creators an unprecedented tool for generating high-quality video sequences. By automating aspects of scene creation, Veo 3 can reduce production times and costs, democratizing access to cinematic storytelling tools. Virtual actors with synchronized audio and realistic facial expressions could become standard, reshaping casting and post-production workflows.
Marketing and advertising sectors stand to benefit by leveraging Veo 3 to create personalized, scalable video ads tailored to individual consumer profiles, thereby enhancing engagement and conversion rates. Brands could also simulate product interactions and scenarios virtually, minimizing the need for expensive physical prototypes.
In education, Veo 3 enables the production of customized training videos and immersive simulations. For example, medical students could experience detailed procedural walkthroughs, and military or industrial trainees could practice in realistic virtual environments. Additionally, Veo 3 could help generate accessible media, such as lifelike sign language interpreters or accurate audio descriptions for those with visual impairments.
AI research and development may harness Veo 3 to create synthetic datasets, particularly valuable in fields such as autonomous vehicles or robotics where real-world data for rare or hazardous scenarios is scarce or expensive to collect.
Market and Business Perspectives: Positioning Veo 3 in the AI Ecosystem
The generative AI market is forecasted to grow exponentially, with a recent report by MarketsandMarkets estimating the global generative AI market will reach $38.7 billion by 2027, up from $6.9 billion in 2022, reflecting a compound annual growth rate of over 40%. Veo 3 positions Google DeepMind strategically within this rapidly expanding market, particularly in the niche of synthetic audiovisual content.
DeepMind’s access to Google’s infrastructure and ecosystem provides a competitive advantage, enabling the deployment of Veo 3 as a cloud-based API accessible to developers, businesses, and creatives worldwide. This model-as-a-service approach opens multiple monetization pathways, including pay-per-use models, enterprise contracts, and partnerships with media and technology companies.
The democratization of cinematic video production empowered by Veo 3 may disrupt traditional creative industries, lowering entry barriers and fostering innovation. However, it also raises questions about market saturation and the potential commoditization of video content.
Ethical Considerations: Balancing Innovation and Responsibility
With the power to create realistic synthetic videos and audio comes responsibility. Veo 3 intensifies concerns surrounding deepfakes, misinformation, and content authenticity. The ability to produce videos indistinguishable from real footage presents risks related to fraud, political manipulation, and erosion of public trust.
To address these concerns, Google DeepMind emphasizes the importance of transparency, watermarking, and detection technologies to help distinguish synthetic media. Industry collaborations and regulatory frameworks will be crucial to mitigate misuse.
Bias in training data remains an ongoing challenge. Despite DeepMind’s efforts to curate diverse datasets, AI systems can unintentionally perpetuate stereotypes or underrepresent marginalized groups. Continuous auditing and improvements are essential.
Computational resource demands also limit the immediate accessibility of Veo 3, raising questions about environmental impact and equitable availability. Efforts to optimize efficiency and extend access to a broader user base are ongoing.
The DeepMind Team Behind Veo 3
Veo 3’s success is the result of the combined expertise of a multidisciplinary team at DeepMind. The project involved specialists in computer vision, audio signal processing, transformer architectures, ethics, and software engineering. Leadership included some of the field’s top researchers in generative models and multimodal learning.
DeepMind fosters a culture of collaboration and innovation, with teams working closely with academic partners, industry stakeholders, and internal ethical review boards. The Veo 3 team exemplifies this approach, iterating rapidly while maintaining rigorous scientific standards and ethical oversight.
Future Directions and Impact
Looking ahead, Veo 3 is a foundation for continued innovation in synthetic media. Research is underway to enable real-time video generation, interactive content creation, and even greater control over stylistic and narrative elements. Integration with virtual and augmented reality platforms is also an anticipated frontier.
As AI-generated video content becomes more prevalent, new norms around creation, consumption, and verification will emerge. Veo 3 not only advances technical capabilities but also catalyzes important societal conversations about the role of synthetic media.
Conclusion
Google DeepMind’s Veo 3 represents a monumental advance in AI-generated video and audio synthesis. Its technical sophistication, grounded in multimodal transformers and hierarchical synthesis, enables the creation of cinematic-quality videos with perfectly synchronized, realistic sound. The model’s development journey showcases years of dedicated research and innovation, supported by extensive datasets and cutting-edge infrastructure.
From entertainment to education, marketing to AI research, Veo 3 opens new horizons for creativity, efficiency, and accessibility. At the same time, it brings challenges that require ethical vigilance and thoughtful governance.
Overall, Veo 3 heralds a new era in artificial intelligence, where the boundaries between real and synthetic media blur—offering powerful tools to augment human creativity while demanding responsibility from creators, platforms, and society at large.